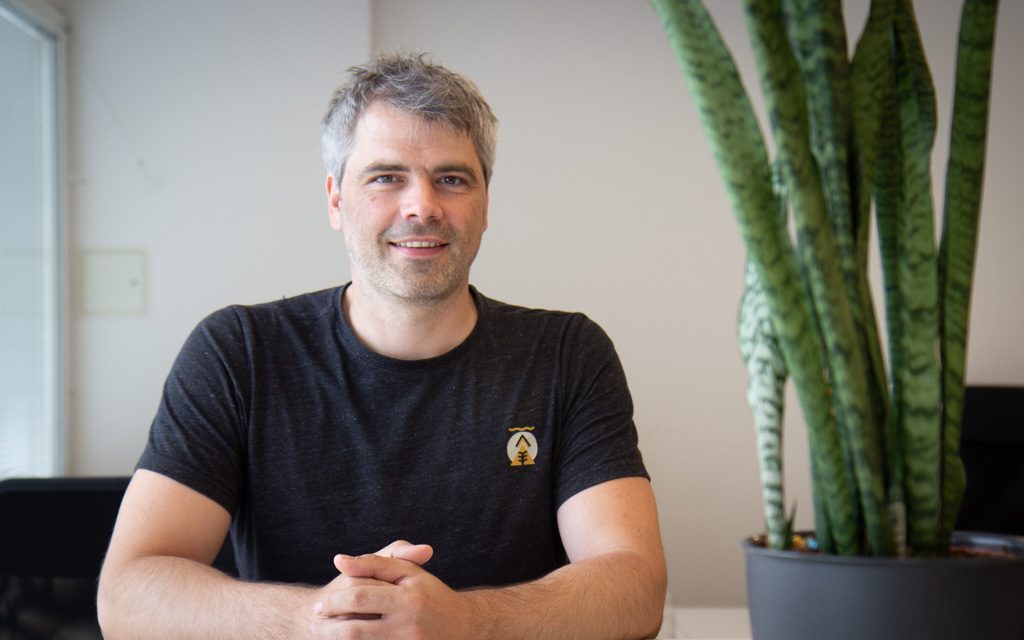
In a previous blog post we explored the potential of predictive analytics to help retailers plan future locations. Now, we lift the hood to find out more about how predictive analysis works and how it can benefit your business. Our guide is David Redlich, team lead for API Services & Data teams at Targomo.
David, simply put, what is predictive analytics?
Imagine if you could predict the performance of future new branches with a high degree of accuracy based on hard facts and not gut feeling. This is what we call predictive analytics. We combine machine learning and geo algorithms as well as socio-demographic, network and performance data to create unique predictive models that help our customers answer questions like: what makes my business successful? How many guests will come to my restaurant? How much revenue can I expect from my outlet store? Where should I expand to next? All of this analysis can now be answered within a fraction of the usual time and effort and with total control and transparency for you.
Before predictive analytics, what did location analysis or planning look like?
Typically, analytics has focused more on sub-aspects and couldn’t handle such large amounts of data. Take consultancies, for example. The analyses are static and usually limited to a few locations. This makes it impossible to run scenarios that take into account the interactions of different locations or look into the future. As the name suggests, predictive analytics are forward-looking. Once the model is in place, customers can perform in-depth analyses on any number of locations without noticeably changing costs. With TargomoLOOP, we have a really nice tool that allows our customers to do their own analysis and get powerful future insights based on data. The platform is scalable as this model can be used worldwide (depending on data availability). We’re confident we’re best in class at predicting a location’s future performance. Plus, we’re continually improving our models and tools.
What kind of data do you use in predictive analysis and where does it come from?
In general, we use four sources: public, private, corporate and self-engineered data.
Publicly-available data includes network data such as transit and traffic information, which we use to create mobility analysis patterns to see how people get to your potential new location. Also, socio-demographic and points-of-interest (POI) data are part of the public data.
Commercial data comprises additional fine-grained socio-demographic data, additional POI data, economic data like spending power or other relevant target group data such as Limbic Types. We obtain these from our reputable worldwide data partners.
An important part of the predictive model is, of course, our customers’ corporate data, meaning location-based data that provides insight into different store characteristics and performance. First and foremost are the sales figures. If we are to develop a predictive model for a specific KPI, we naturally need the data from the existing stores. Also, information such as different closing times, sales areas, etc are also important in order to examine their impact or to be able to explain deviations.
Another relevant source that differentiates us from our competitors is successfully engineered domain-specific data. We provide foot traffic broken down by time of day and night, and on intentions such as shopping or restaurant visits. We offer car or home ownership ratios and custom POI sets that are particularly interesting brick-and-mortar businesses to see what complimentary businesses or services are around their sites.
What are some of the challenges in creating an accurate predictive analytics model?
At the beginning of a project, a considerable amount of time still goes into collecting, scraping and cleansing the data. This step is often underestimated but crucial in order to provide our customers with reliable results. The main challenge, however, is that AI and machine learning algorithms are usually not optimised for geo-spatial data (geo-information, POIs, network data and store locations). Getting those things to fit together is challenging. One of the things we did to solve that is the usage of established economical models – like the gravitational or logit models. In these models, all locations have an “attraction” with which you can calculate the probabilities of certain demographics coming to your place, taking into account other competing locations and their attraction. The complexity of the problem is incredible. Many people have tried to solve this problem with different levels of success. But, I believe, none have managed to in the generalised manner we have. Our predictive analytics works across domains. You can just plug in the data and find individual success drivers without any domain-specific or company-specific customisation. That is a pretty big challenge we overcame.
How does TargomoLOOP address customers’ needs around predictive analytics?
One key influence for us is the user experience. For instance, we want to put TargomoLOOP entirely into the hands of our customers. We don’t want to act as the middleman doing the analysing for them. We want to give them a tool with which they can play through many different scenarios by themselves. It’s a fine balance – and we’re still learning how to communicate complex results but at the same time give our customers a lot of user control. We have always worked a lot with test users and collect feedback from existing customers in order to achieve a good user experience and to develop it further.
What is the role of the client in predictive analytics?
Customers play an important role before and during development. At the beginning, they provide us with the store and company data (e.g. KPIs) as well as environment-independent store facts.
They then also have an active role during the evaluation. When we have the initial results on the success drivers, we run the results past them to see if it is comprehensible to them. It’s usually not a complete surprise what the success drivers are, but to what extent they are important. After all, we quantify exactly how important car and pedestrian traffic are on site and what role competitors play. After the analysis, they know how much of their sales are based on each factor. Sometimes we also find out that factors that were seen as important really have no impact on sales at all.
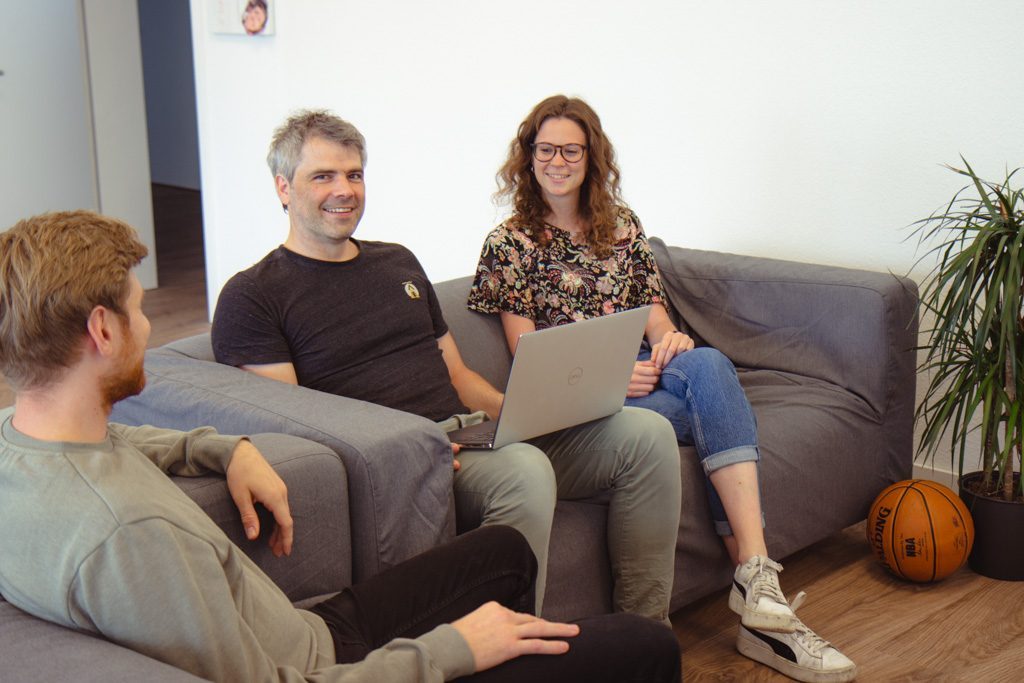
Predictive Analytics is always team effort: David and team discussing the analytics results.
What are the skills needed to create predictive analytics models?
It has been a steep learning curve for us and we created a research project together with universities to draw in knowledge that we didn’t have. We also expanded our teams with very capable data scientists. Together with our great software developers we created a successful symbiosis to offer cutting edge Geo AI as an enterprise product. I wouldn’t limit the needed skill set to that of data scientists or software developers, though. Marketing, business development and customer success are very important as well. It’s crucial the concepts and results are communicated in an understandable manner. That’s a difficult task.
David, thank you for taking us through predictive analysis. While none of us possess a crystal ball, as we’ve seen with predictive analysis, it really can help predict future outcomes in order to successfully plan retail locations and minimize risks. And the best bit is, the tool is intuitive to use, allowing our clients to plug-and-play to find the scenarios that work best for them.
Comments